Invite-Only AI Research Presentation Furthers Possibility of Apple Branded Autonomous Driving Technology
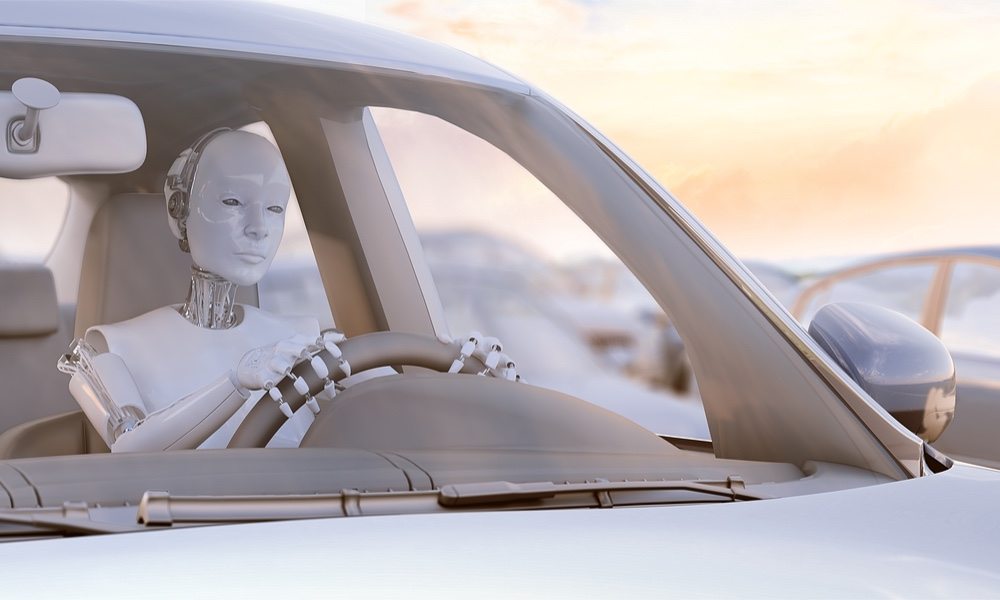
Toggle Dark Mode
Artificial intelligence has been making headlines recently as the investments keep pouring in and companies race to develop their own neural networks and machine learning capabilities. One tech giant that has been relatively tight-lipped about its AI ambitions has been Apple, which has a reputation for zealously guarding its secrets.
Recently, Cupertino opened up at an AI industry conference, at which it hosted an invite-only luncheon led by Russ Salakhutdinov, Apple’s head of machine learning. It is the most comprehensive overview of Apple’s machine learning research and ambitions we’ve received to date.
The presentation was detailed in a new Quartz report, which reveals that some of the issues that Salakhutdinov’s team are working on include image recognition, language modeling, dealing with uncertainty, and predicting user behavior — which are fairly popular areas of machine learning research. Headway on such issues would lead to noticeable performance gains for Siri, as Apple prepares to compete with the likes of Amazon and Google to bring the best personal AI assistant to consumers.
Another presentation slide, titled “Volumetric Detection of LiDAR”, suggests that Apple is working to develop its self-driving technology. LiDAR, or Light Detection and Radar, is a crucial component of self-driving cars that uses lasers to detect its surroundings. This tidbit, combined with Apple’s recent letter to the NHTSA, further solidifies the view that the company’s Project Titan-successor definitely fits square within the burgeoning autonomous transport sector.
In other slides, Apple boasted about the strides it had already made in neural networks. It claimed that its GPU-based image processing network could process twice as many photos per second as Google’s, while leveraging only one-third as many GPUs.
Salakhutdinov also announced that the team had developed neural networks that are 4.5 times smaller and twice as fast as the originals, without sacrificing accuracy. This is a promising development in a technique known as teacher-student neural networks, where a smaller “student” network learns from a much larger “teacher” network. Streamlined student networks could then be implemented on small devices like, say, iPhones without requiring as much processing power as the teacher. This is also a faster and more secure option than having iPhones offload image and facial recognition processing to remote servers and encrypting the wireless data transfers.