Apple Explains the Challenges of ‘On-Device’ Facial Recognition
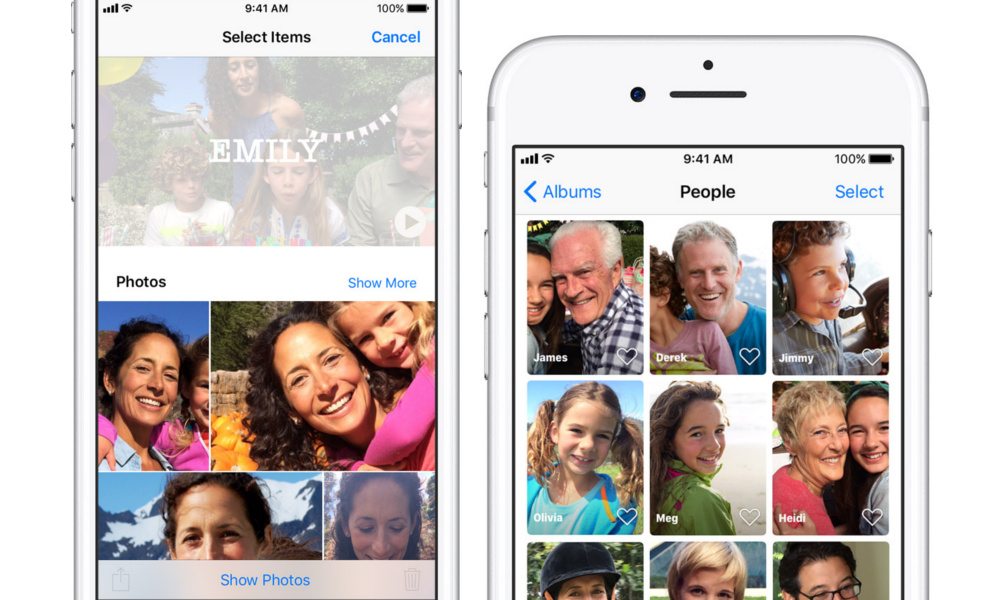
Toggle Dark Mode
Apple has published a new entry in its Machine Learning Journal detailing the challenges of powering facial recognition features with deep neural networks while at the same time protecting user privacy.
The iPhone maker first integrated deep learning algorithms into face detection with the introduction of the Core Image framework in iOS 10. Facial recognition technology was used to identify faces in photos so that users could organize and view them by person in the Photos app. In the weeks following the release of the iPhone X, facial recognition algorithms have come to play an even more prominent role in the everyday lives of Apple users.
And, with the launch of Apple’s Vision framework, which allows Apple developers to use those computer vision algorithms in their apps, the challenge has become a matter of maintaining user privacy while allowing face detection to run efficiently.
Due to Apple’s commitment to user privacy and security, running those computer vision algorithms on an iCloud server was not an option.
“Apple’s iCloud Photo Library is a cloud-based solution for photo and video storage. However, due to Apple’s strong commitment to user privacy, we couldn’t use iCloud servers for computer vision computations. Every photo and video sent to iCloud Photo Library is encrypted on the device before it is sent to cloud storage, and can only be decrypted by devices that are registered with the iCloud account. Therefore, to bring deep learning based computer vision solutions to our customers, we had to address directly the challenges of getting deep learning algorithms running on iPhone.”
Eventually, Apple figured out a way to run face detection locally and efficiently on devices, which is no mean feat given the amount of processing power and storage space deep-learning models require. That entailed configuring an optimal framework that would fully leverage the on-device GPU and CPU, as well as memory use.
“The deep-learning models need to be shipped as part of the operating system, taking up valuable NAND storage space,” Apple wrote. “They also need to be loaded into RAM and require significant computational time on the GPU and/or CPU. Unlike cloud-based services, whose resources can be dedicated solely to a vision problem, on-device computation must take place while sharing these system resources with other running applications. Finally, the computation must be efficient enough to process a large Photos library in a reasonably short amount of time, but without significant power usage or thermal increase.”